Pilot project 3 – Autonomous shipping technology supported by AI
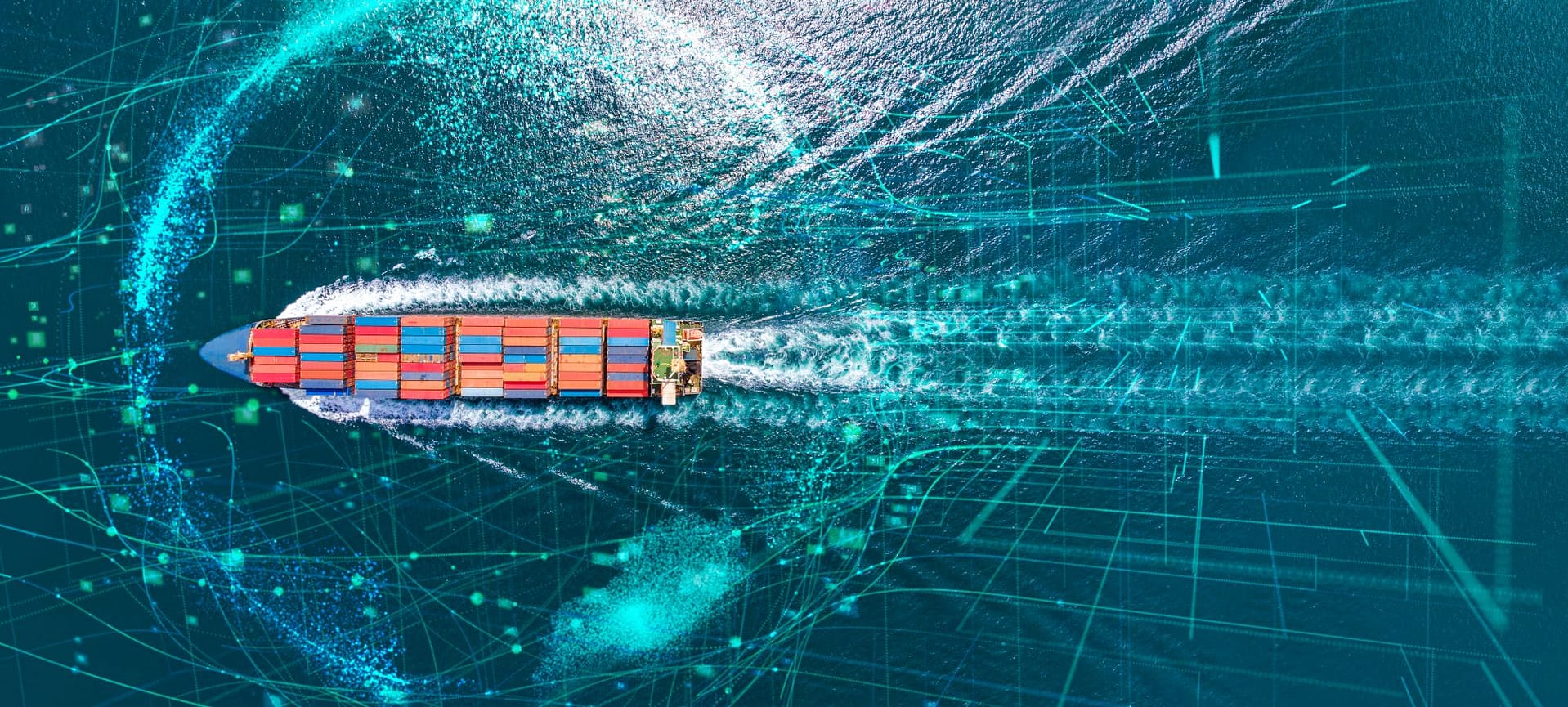
CHALLENGE! To build a model for the automatic detection of potentially reckless and inexperienced personal watercraft drivers, monitor rental boats, and predict the driving behavior of motorboat operators.
HOW? Detecting trajectory anomalies using inflection point sequences, and using a Bayesian, Markov chain, and different machine learning models for forecasting.
WHY? To provide the necessary information to avoid collisions and hazards, especially in crowded spaces during the peak of the tourist season in crowded or narrow areas.
FINAL RESULT→ Anomaly detection, and forecasting models for small personal vessels.
GOALS FOR INNO2MARE PROJECT: To assist in creating a new speed-limiting algorithm for small personal watercraft operators that could replace the existing proximity-based approach.
Physical Setup and Prototyping
The infrastructure, facility and equipment from the Croatian partners are located at UNIRI RITEH (Faculty of Engineering, Vukovarska 58, 51000 Rijeka, Croatia) and UNIRI FIDIT (Faculty of Informatics and Digital Technologies, Ul. Radmile Matejcic 2, 51000, Rijeka, Croatia).
The setup includes:
- PC workstation for machine learning and AI, other hardware and software (UNIRI RITEH, UNIRI FIDIT, laboratories).
- Sensors and other equipment for data acquisition, Belgium partners to assure additional datasets for ML approaches, their equipment located on ships, ports.
- Autonomous shipping supported by high-performance computing (HPC) and advanced AI algorithms.
Recent Developments and Progress
Pilot progress 3 until 30.6.2025:Personal Watercraft Trajectory Prediction and Classification
CHALLENGE! To build a model for the automatic detection of potentially reckless and inexperienced personal watercraft drivers, monitor rental boats, and predict the driving behavior of motorboat operators.
HOW? Detecting trajectory anomalies using inflection point sequences, and using a Bayesian, Markov chain, and different machine learning models for forecasting.
WHY? To provide the necessary information to avoid collisions and hazards, especially in crowded spaces during the peak of the tourist season in crowded or narrow areas.
FINAL RESULT→ Anomaly detection, and forecasting models for small personal vessels.
GOALS FOR INNO2MARE PROJECT: To assist in creating a new speed-limiting algorithm
for small personal watercraft operators that could replace the existing proximity-based approach.
Progress
Experiments and actions on the pilot project so far:
1. Using inflection points for trajectory clustering and anomaly detection
We developed a novel trajectory fingerprinting method using inflection point encoding, translating trajectory direction changes into hexadecimal-coded fingerprints. These were clustered using DBSCAN and K-means. The method showed over 85% alignment with Intelligent Distance Control (IDC) labels and expert judgment, confirming its effectiveness for real-time anomaly detection in small personal watercraft. The fingerprinting approach is lightweight, real-time-compatible, and significantly more efficient than deep learning models.Gathering real-world training data was essential for all the described models. However, we also filtered the data to exclude missing transmissions (“ERROR” flagged entries), and included interpolation in cases where large gaps in transmission were observed in the input.
Below are the key activities implemented thus far in assembling an anomaly detection model:
Dataset Expansion: We merged data for 6911 rides from 1282 locations, and 5345 vehicles in Europe, Africa, Asia, Australia, North America, and South America, recorded from November 12, 2021 to February 21, 2025. We also analyzed an AIS dataset collected near Brest, France, including static and dynamic information received for tankers, cargo vessels, passenger vessels, fishing boats, pleasure crafts, rowing and tugboats, military vessels, and other types of vessels, spanning from October 1, 2015, to March 31, 2016.
Conducting a Human Similar Trajectory Selection Experiment: Experts manually selected 5 out of the 20 trajectories in a web interface based on the perceived similarity to a baseline trajectory.
Anomaly Validation: We validated DBSCAN and k-Means clustering based on inflection point sequences with real-world OtoTrak data on Intelligent Distance Control (IDC) activation, and with AIS data using non-zero rate of turn (ROT).
This helped us develop a robust, location-agnostic framework for forecasting and anomaly detection based on the movement of personal watercraft and larger vessels, enhancing maritime safety, reducing risks in crowded environments, and improving decision-making in semi-autonomous and remote-control navigation systems. Tourist-heavy coastal and freshwater rental locations present complex navigation challenges due to inexperienced operators and unpredictable behavior. Forecasting future trajectories of personal watercraft allows the avoidance of unnecessary speed limits and enhances safety. Expert feedback, algorithm selection, and clustering were compared to IDC control labels to validate the generated classification. The proposed clustering algorithms provide a basis for anomaly detection in cases where labels are not available beforehand.
2. A Bayesian and Markov chain approach to short-term and long-term personal watercraft trajectory forecasting
We developed Bayesian, and Markov chain approaches to long-, and short-term trajectory forecasting without machine learning using several approaches:
- A Bayesian, and Markov chain approach using one or two previous states;
- A Bayesian, and Markov chain approach using one or two previous states, and conditional probability dependent on wave height;
- A Bayesian, and Markov chain approach using one or two previous states, and conditional probability dependent on wind speed;
- A Bayesian, and Markov chain approach using one or two previous states, and conditional probability dependent on temperature.
Forecasting targets included speed, heading, and latitude and longitude offsets. Trajectory reconstruction was done directly using predicted latitude and longitude offset values or derived using speed, heading, and time intervals in trigonometric calculations. A Bayesian approach with two previous actual values achieved promising results in single-step forecasting. This confirms its practical utility for real-time control, especially in embedded systems. Markov chains were formalized with ergodic properties and transition probabilities, supporting robust stochastic modeling. These methods present a strong foundation for behavior-aware speed limitation systems.
3. Forecasting the trajectory of personal watercrafts using models based on recurrent neural networks
Tourist-heavy coastal and freshwater rental locations present complex navigation challenges due to inexperienced operators and unpredictable behavior. Forecasting future trajectories of personal watercraft allows the avoidance of unnecessary speed limits and enhances safety:
- Recurrent neural network (RNN) models with simple RNN, long short-term memory (LSTM), or gated recurrent unit (GRU) layers in four architectures – forecasting the trajectories of automobiles on highways;
- A GRU attention model architecture with four experiment settings – used for sequence-to-sequence translation, adapted to process numbers;
- LSTM bidirectional and convolutional models for peptide self-assembly – adapted for prediction instead of classification, processing trajectories instead of sequential properties, and aggregation propensity;
- The Unified Time Series Model (UniTS) model – developed for use on diverse time-series data, and tasks, without retraining, but adapted to our data using zero-shot.
Preprocessing techniques included the normalization and scaling of all variables, latitude and longitude converted to relative offsets, and trajectories mirrored for directional consistency. The UniTS foundation model achieved the best overall performance, especially in long-term forecasting, and can generalize across locations without retraining (zero-shot learning). The GRU attention models performed best in short-term forecasting of trajectory offsets. Traditional models like Bayesian and Markov chains underperformed compared to neural approaches. Future steps include integrating forecasting model into real-time maritime tracking systems to reduce false positive collision detection and unnecessary IDC triggers. This will enable the use of smarter speed-limiting protocols that respond to predicted, not just current, behavior, and enhance modular systems for remote control and semi-autonomous vessel operation. We plan to further refine models for latency tolerance and robustness in low-connectivity environment and develop a predictive system to replace traditional proximity-based speed limitations. This will contribute to maritime traffic safety innovations and the digitalization of marine transport monitoring.
Papers to be cited:
Lucija Žužić, Ivan Dražić, Loredana Simčić, Franko Hržić, Jonatan Lerga, A Bayesian and Markov chain approach to short-term and long-term personal watercraft trajectory forecasting, Journal of the Franklin Institute, Volume 362, Issue 3, 2025, 107509,
ISSN 0016-0032, https://doi.org/10.1016/j.jfranklin.2025.107509.
Lucija Žužić, Franko Hržić, Jonatan Lerga, Forecasting the trajectory of personal watercrafts using models based on recurrent neural networks, Expert Systems with Applications, Volume 284, 2025, 127964, ISSN 0957-4174, https://doi.org/10.1016/j.eswa.2025.127964.
Progress 30.6.2023
Progress 31.12.2023
Progress 30.6.2024
Progress 31.12.2024
Progress 30.6.2025
Lesson learnt and implementation strategy
Publications
Your Title Goes Here
Your content goes here. Edit or remove this text inline or in the module Content settings. You can also style every aspect of this content in the module Design settings and even apply custom CSS to this text in the module Advanced settings.
Estimation of sea state parameters from ship motion responses using attention-based neural networks
Denis Selimović, Franko Hržić, Jasna Prpić-Oršić, Jonatan Lerga, Estimation of sea state parameters from ship motion responses using attention-based neural networks, Ocean Engineering, Volume 281, 2023.
https://arxiv.org/abs/2301.08949
Abstract: On-site estimation of sea state parameters is crucial for ship navigation. Extensive research has been conducted on model-based estimation utilizing ship motion responses. Model-free approaches based on machine learning (ML) have recently gained popularity, and estimation from time-series of ship motion responses using deep learning (DL) methods has given promising results. In this study, we apply the novel, attention-based neural network (AT-NN) for estimating wave height, zero-crossing period, and relative wave direction from raw time-series data of ship pitch, heave, and roll. Despite reduced input data, it has been demonstrated that the proposed approaches by modified state-of-the-art techniques (based on convolutional neural networks (CNN) for regression, multivariate long short-term memory CNN, and sliding puzzle neural network) improved estimation MSE, MAE, and NSE by up to 86%, 66%, and 56%, respectively, compared to the best performing original methods for all sea state parameters. Furthermore, the proposed technique based on AT-NN outperformed all tested methods (original and enhanced), improving estimation MSE by 94%, MAE by 74%, and NSE by 80% when considering all sea state parameters. Finally, we proposed a novel approach for interpreting the uncertainty estimation of neural network outputs based on the Monte-Carlo dropout method to enhance the model’s trustworthiness.
Keywords: Ship motions; Sea state estimation; Deep learning; Attention neural network; Uncertainty estimation
Application of raycast method for person geolocalization and distance determination using UAV images in Real-World land search and rescue scenarios
Goran Paulin, Sasa Sambolek, Marina Ivasic-Kos, Application of raycast method for person geolocalization and distance determination using UAV images in Real-World land search and rescue scenarios, Expert Systems with Applications, Volume 237, Part A, 1 March 2024, 121495.
https://papers.ssrn.com/sol3/papers.cfm?abstract_id=4450690
Abstract: People enjoy spending time in the wilderness for numerous reasons. However, they occasionally get lost or injured, and their survival depends on being efficiently found and rescued in the shortest possible time. A search and rescue operation (SAR) is launched after the accident is reported, and all possible resources are activated. The inclusion of drones in SAR operations has enabled the use of computer vision methods to detect persons in aerial imagery automatically. When searching by drone, preference is given to oblique photographs that cover a larger area within a single image, reducing the search time. Unlike vertical photographs, oblique photographs include a significant scale change, making it challenging to locate a person in the real world and determine their distance from the drone. In order to solve this problem, encouraged by our previous successful simulations, we explored the possibility of applying the raycast method for person geolocalization and distance determination for use in real-world scenarios. In this paper, we propose a system able to precisely geolocate persons automatically detected in offline processed images recorded during the SAR mission. After a series of experiments on terrains of different configurations and complexity, using a custom-made 3D terrain generator and raycaster, along with a deep neural network-based person detector trained on our custom dataset, we defined a method for geolocating detected person based on raycast, which allows using low-cost commercial drones with a monocular camera and no Real-Time Kinematic module while enabling laser rangefinder emulation during offline image analysis. Our person geolocating method overcomes the problems faced by previous methods and, using a single flight sequence with only 4 consecutive detections, significantly outperforms the previous best results, with reliability of 42,85% (geolocating error of 0.7 m on recording from a 30 m height). Also, a short time of only 247 s enables offline processing of data recorded during a 21-minute drone flight covering approximately an area of 10 ha, proving that the proposed method can be effectively used in actual SAR missions. We also proposed a new evaluation metric (ErrDist) for person geolocalization and provided recommendations for using the proposed system for person detection and geolocation in real-world scenarios.
Keywords: Raycasting; Drone imagery; Object detection; YOLOv4; Object geolocalization; Distance determination; Search and rescue missions
Detection of motor imagery based on short-term entropy of time-frequency representations
Luka, Batistić; Jonatan, Lerga; Isidora, Stanković , Detection of motor imagery based on short-term entropy of time-frequency representations, BioMedical Engineering OnLine volume 22, Article number: 41 (2023)
https://doi.org/10.1186/s12938-023-01102-1
Abstract:
Motor imagery is a cognitive process of imagining a performance of a motor task without employing the actual movement of muscles. It is often used in rehabilitation and utilized in assistive technologies to control a brain–computer interface (BCI). This paper provides a comparison of different time–frequency representations (TFR) and their Rényi and Shannon entropies for sensorimotor rhythm (SMR) based motor imagery control signals in electroencephalographic (EEG) data. The motor imagery task was guided by visual guidance, visual and vibrotactile (somatosensory) guidance or visual cue only.
When using TFR-based entropy features as an input for classification of different interaction intentions, higher accuracies were achieved (up to 99.87%) in comparison to regular time-series amplitude features (for which accuracy was up to 85.91%), which is an increase when compared to existing methods. In particular, the highest accuracy was achieved for the classification of the motor imagery versus the baseline (rest state) when using Shannon entropy with Reassigned Pseudo Wigner–Ville time–frequency representation.
Our findings suggest that the quantity of useful classifiable motor imagery information (entropy output) changes during the period of motor imagery in comparison to baseline period; as a result, there is an increase in the accuracy and F1 score of classification when using entropy features in comparison to the accuracy and the F1 of classification when using amplitude features, hence, it is manifested as an improvement of the ability to detect motor imagery.
Keywords: Brain–computer interface , Electroencephalography , Information entropy, Motor imagery, Movement detection , Time–frequency representations
Evaluating YOLOV5, YOLOV6, YOLOV7, and YOLOV8 in Underwater Environment: Is There Real Improvement?
Boris Gašparović; Goran Mauša; Josip Rukavina; Jonatan Lerga, Evaluating YOLOV5, YOLOV6, YOLOV7, and YOLOV8 in Underwater Environment: Is There Real Improvement?
DOI: https://papers.ssrn.com/sol3/papers.cfm?abstract_id=5156721
Published in: 2023 8th International Conference on Smart and Sustainable Technologies (SpliTech)
Abstract:
This paper compares several new implementations of the YOLO (You Only Look Once) object detection algorithms in harsh underwater environments. Using a dataset collected by a remotely operated vehicle (ROV), we evaluated the performance of YOLOv5, YOLOv6, YOLOv7, and YOLOv8 in detecting objects in challenging underwater conditions. We aimed to determine whether newer YOLO versions are superior to older ones and how much, in terms of object detection performance, for our underwater pipeline dataset. According to our findings, YOLOv5 achieved the highest mean Average Precision (MAP) score, followed by YOLOv7 and YOLOv6. When examining the precision-recall curves, YOLOv5 and YOLOv7 displayed the highest precision and recall values, respectively. Our comparison of the obtained results to those of our previous work using YOLOv4 demonstrates that each version of YOLO detectors provide significant improvement.
Author Keywords: object detection, yolov5, yolov6, yolo7, yolov8, comparison
A computer vision approach to estimate the localized sea state
Aleksandar Vorkapic, Miran Pobar, Marina Ivasic-Kos, A computer vision approach to estimate the localized sea state, Ocean Engineering , Volume 309, Part 1, 1 October 2024, 118318.
https://arxiv.org/abs/2407.03755
Abstract
This research presents a novel application of computer vision (CV) and deep learning methods for real-time sea state recognition, aiming to contribute to improving the operational safety and energy efficiency of seagoing vessels, key factors in meeting the legislative carbon reduction targets. Our work focuses on utilizing sea images in operational envelopes captured by a single stationary camera mounted on the ship bridge. The collected images are used to train a deep learning model to automatically recognize the state of the sea based on the Beaufort scale. To recognize the sea state, we used 4 state-of-the-art deep neural networks with different characteristics that proved useful in various computer vision tasks: Resnet-101, NASNet, MobileNet_v2, and Transformer ViT -b32. Furthermore, we have defined a unique large-scale dataset, collected over a broad range of sea conditions from an ocean-going vessel prepared for machine learning. We used the transfer learning approach to fine-tune the models on our dataset. The obtained results demonstrate the potential for this approach to complement traditional methods, particularly where in-situ measurements are unfeasible or interpolated weather buoy data is insufficiently accurate. This study sets the groundwork for further development of sea state classification models to address recognized gaps in maritime research and enable safer and more efficient maritime operations.
Keywords: Energy efficient shipping, Computer vision, Sea state recognition, Deep neural networks, Real-time monitoring
Interpretable Machine Learning: A Case Study on Predicting Fuel Consumption in VLGC Ship Propulsion
Aleksandar Vorkapić, Sanda Martinčić-Ipšić, Rok Piltaver, Interpretable Machine Learning: A Case Study on Predicting Fuel Consumption in VLGC Ship Propulsion, Journal of Marine Science and Engineering, 2024, 12(10),1849.
https://doi.org/10.3390/jmse12101849
Abstract
The integration of machine learning (ML) in marine engineering has been increasingly subjected to stringent regulatory scrutiny. While environmental regulations aim to reduce harmful emissions and energy consumption, there is also a growing demand for the interpretability of ML models to ensure their reliability and adherence to safety standards. This research highlights the need to develop models that are both transparent and comprehensible to domain experts and regulatory bodies. This paper underscores the importance of transparency in machine learning through a use case involving a VLGC ship two-stroke propulsion engine. By adhering to the CRISP-DM standard, we fostered close collaboration between marine engineers and machine learning experts to circumvent the common pitfalls of automated ML. The methodology included comprehensive data exploration, cleaning, and verification, followed by feature selection and training of linear regression and decision tree models that are not only transparent but also highly interpretable. The linear model achieved an RMSE of 23.16 and an MRAE of 14.7%, while the accuracy of decision trees ranged between 96.4% and 97.69%. This study demonstrates that machine learning models for predicting propulsion engine fuel consumption can be interpretable, adhering to regulatory requirements, while still achieving adequate predictive performance.
Keywords: interpretability; machine learning; decision trees; linear regression; feature selection; two-stroke marine engines; fuel consumption
A Bayesian and Markov chain approach to short-term and long-term personal watercraft trajectory forecasting
A Bayesian and Markov chain approach to short-term and long-term personal watercraft trajectory forecasting
Lucija Žužić , Ivan Dražić , Loredana Simčić , Franko Hržić , Jonatan Lerga , A Bayesian and Markov chain approach to short-term and long-term personal watercraft trajectory forecasting, Journal of the Franklin Institute , January 2025.
https://papers.ssrn.com/sol3/papers.cfm?abstract_id=5156719
(https://www.sciencedirect.com/science/article/pii/S0016003225000031)
Abstract:
In this work, vessel position is estimated using a Bayesian approach based on heading, speed, time intervals, and offsets of latitude and longitude. An additional approach using a Markov chain is presented. The trajectory data comes from a cloud-based marine watercraft tracking system that enables remote control of the vessels. Wave height and meteorological reports were used to evaluate the impact of weather on personal watercraft trajectories. One proposed approach to trajectory estimation uses the longitude and latitude offsets, while another uses the speed, heading, and actual time intervals. A long-term forecasting window of up to ten seconds is achieved by dividing trajectories into segments that do not overlap. The limitation this method faces in long-term forecasting inspires more sophisticated machine-learning approaches. The most successful estimation method used one or two previous actual values and a Bayesian approach, proving that using previously predicted values in a chain accumulates errors. Considering environmental variables did not improve the model, highlighting that small watercrafts operate well even in unstable sea states. This occurs because they generate and ride waves, having a larger impact than oceanic currents.
Keywords
Personal watercraft, Trajectory forecasting, Markov chain
A comprehensive review of datasets and deep learning techniques for vision in unmanned surface vehicles
A comprehensive review of datasets and deep learning techniques for vision in unmanned surface vehicles
Linh Trinh, Siegfried Mercelis, Ali Anwar , A comprehensive review of datasets and deep learning techniques for vision in unmanned surface vehicles, Ocean Engineering , Volume 334, 1 August 2025, 121501
https://doi.org/10.1016/j.oceaneng.2025.121501
Abstract:
Unmanned Surface Vehicles (USVs) have emerged as a major platform in maritime operations, capable of supporting a wide range of applications. USVs allow for difficult unmanned tasks in harsh maritime environments. With the rapid development of USVs, many vision tasks such as detection and segmentation become increasingly important. Datasets play an important role in encouraging and improving the research and development of reliable vision algorithms for USVs. In this regard, a large number of recent studies have focused on the release of vision datasets for USVs. Along with the development of datasets, a variety of deep learning techniques have also been studied, with a focus on USVs. However, there is a lack of a systematic review of recent studies in both datasets and vision techniques to provide a comprehensive picture of the current development of vision on USVs, including limitations and trends. In this study, we provide a comprehensive review of both USV datasets and deep learning techniques for vision tasks. Our review was conducted using a large number of vision datasets from USVs. We elaborate several challenges and potential opportunities for research and development in USV vision based on a thorough analysis of current datasets and deep learning techniques.
Keywords
Unmanned surface vessels; Datasets; Deep learning; Computer vision
Forecasting the trajectory of personal watercrafts using models based on recurrent neural networks
Lucija Žužić , Franko Hržić , Jonatan Lerga; Forecasting the trajectory of personal watercrafts using models based on recurrent neural networks, Expert Systems with Applications, Volume 284, 25 July 2025, 127964
https://doi.org/10.1016/j.eswa.2025.127964
Abstract:
Monitoring and predicting personal watercraft trajectories is a novel and largely unexplored research area where any development is valuable for various rental services. Unlike existing work focused on specific maritime routes, this study introduces a location-agnostic deep-learning approach capable of generalizing across diverse environments. This is achieved by using an innovative preprocessing approach including offset and scaling. A novel Long Short-Term Memory (LSTM) bidirectional and convolutional model developed by the authors for binary peptide classification was adapted to accommodate the forecasting of continuous values. By leveraging Recurrent Neural Network (RNN) architectures with LSTM and Gated Recurrent Unit (GRU) layers, and cutting-edge attention-based and foundation models, we benchmark trajectory forecasting performance using real-world data from 1282 rental sites worldwide. Most notably, we extend the applicability of the listed models and the original LSTM bidirectional and convolutional models to maritime trajectory estimation, eliminating the need for training separate models for different locations while achieving superior predictive accuracy. Our results demonstrate that foundation models and attention mechanisms significantly outperform traditional methods, offering a robust and scalable solution for watercraft trajectory forecasting. These findings pave the way for intelligent monitoring systems that enhance maritime safety and operational efficiency.
Keywords: Personal watercraft, Trajectory forecasting, Recurrent neural network , Attention mechanism , Foundation model